An artificial intelligence tells Google how to design better chips
Google is using artificial intelligence to design chips that accelerate artificial intelligence. It looks like a play on words, but it is. Through a reinforcement learning algorithm, it is possible to create faster and more efficient projects in less time.
Google has created a new ” reinforcement learning ” algorithm, which has autonomously learned how to create more powerful and fewer energy-hungry processors dedicated to artificial intelligence (TPU). The algorithm was able to improve the arrangement on the chip of the various components and the related interconnections.
Creating a processor of any kind is not an easy task at all. The positioning of the various logical and memory blocks, also known as ” chip floor planning, “presents a very complex design problem, as a careful configuration of hundreds, if not thousands, of blocks placed on different layers in a limited area.
As a rule, engineers work primarily ” manually, “thinking of configurations to reduce the number of connections between components to improve efficiency. Then software that automates the design to simulate and verify performance are put to work. This phase can take up to 30 hours for a single layer.
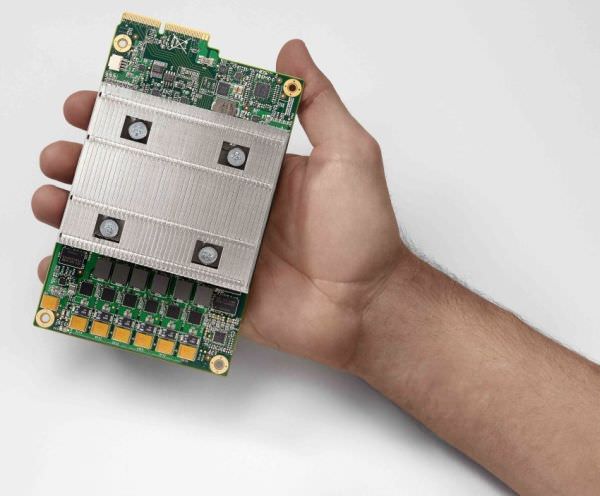
The time to design a better chip is not only long, but there is another problem that companies and engineers are facing. Usually, the chips created should last from two to five years, but the rapid advancement of machine learning algorithms it is accelerating the need for new computing architectures in shorter times.
In recent years, several algorithms have been created to optimize the arrangement of the components. Still, they have a limit: they are unable to follow multiple objectives, including energy consumption, calculation performance, and the occupied area.
” We believe that artificial intelligence itself must provide the means to shorten the chip design cycle, creating a symbiotic relationship between hardware and AI, with each fueling progress in the other, ” reads a paper published by Google on ArXiv.
” We have already seen that there are algorithms or architectures of neural networks that … do not work as well on the generations of existing accelerators, because the accelerators were designed with the knowledge of two years ago, and at the time these neural networks did not exist. If we reduce the design cycle, we can close the gap. ”
Google researchers Anna Goldie and Azalia Mirhoseini have thus chosen to follow a new approach, reinforced learning. Unlike deep learning, these algorithms are not trained through a large set of labeled data, but ” learn by doing, “adjusting the parameters in their networks based on a ” reward ” signal that is given to them when they are successful.
The algorithm then processed hundreds to thousands of new projects, each within a fraction of a second (in total, it took less than 24 hours), evaluating them using the reward function. Over time, the algorithm has thus come up with a final strategy for optimally arranging the components. By analyzing the designs created, Google found that many choices made by the algorithm were better than those made by human engineers.
The hope of the Mountain View house is that this algorithm will speed up the development of better projects – capable of higher performance, lower consumption, and less expensive to produce – to accelerate the creation of ever more advanced real applications in the field of artificial intelligence.